Preparing for a Mass Patient Move
How the University of Texas Medical Branch predicted an effective relocation for 185 patients
Objectives
- Troubleshoot potential problems
- Evaluate delay times and bottlenecks
- Optimize staffing hours
- Observe how one unit’s delay would affect other units
Results
- Discovered the optimal number of move teams (5)
- Predicted end times for the move based on scenarios with varying delay probabilities
- Saved time for 15-25 move staff and additional hospital staff by finishing early
- Now have a skeleton model for future scenarios
Would you like a PDF copy of the case study?

Moving patients comes with uncertainties, as any healthcare administrator knows. Safety—in 2013, one US-based health system reported that 219 patients fell while being transported across its hospitals and clinics—must be balanced with the right staff and procedure for the task; this requires precision and careful planning. On one particular Saturday, the University of Texas Medical Branch (UTMB) relocated 185 patients from an existing hospital to a new, adjacent construction more than 1,200 feet away. UTMB sought to create a safe and efficient moving experience by combining systems engineering principles and methods with discrete event simulation.
To help with this task, UTMB chose FlexSim HC to create a 3D simulation model of the move conditions. Building the model helped them identify any potential problems that could occur during the move and allowed them to study possible solutions to these problems—all within a risk-free virtual environment. The model also helped them determine the optimal number of staff members needed for the move and predict how long the move would actually take.
Solving problems before they arise
Since the completion time could vary based on delays, UTMB considered a wide range of impediments that could be experienced during the move. Using data gathered from several practice moves, they were able to see delays and bottlenecks at every stage in the journey, and even see how delay in one unit would affect other units and the total move time. What did they learn? The move could be completed in six hours and five minutes with no delays, with the model predicting alternate finish times based on several delayed scenarios.
Maximizing valuable human resources
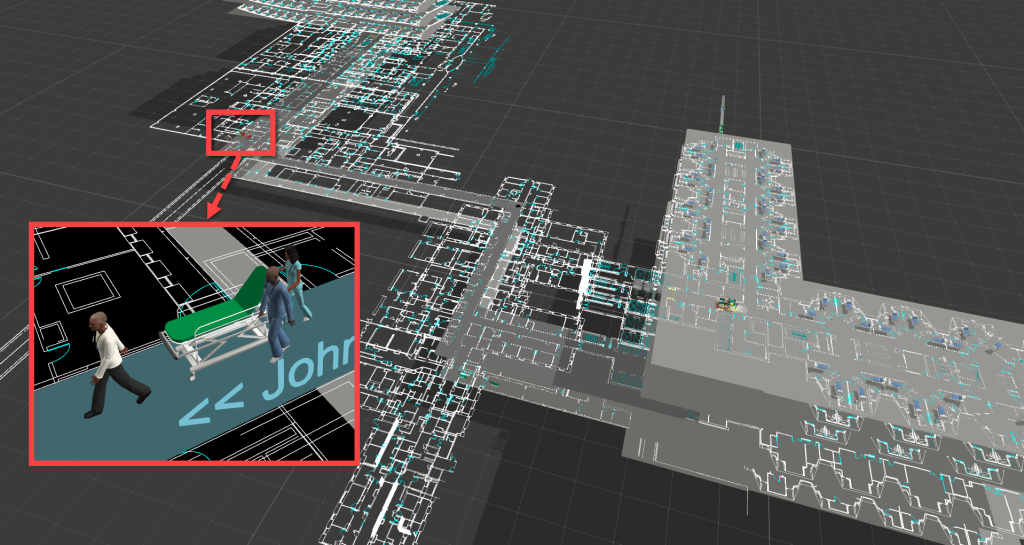
Since staff time is the main resource UTMB would expend during the move, it was critical to find the right number of move teams. These teams consisted of transportation and equipment staff led by either a physician and a nurse (for critical patients) or two nurses (for non-critical patients). UTMB experimented with different configurations and found that five teams were ideal for the situation. Simulations run in FlexSim HC showed that, while four teams could have handled the move, five teams ensured there would be no down time.
Predicting a successful outcome
The actual move started at 7:00 AM and was completed at 1:35 PM, just six hours and thirty-five minutes from when it began. This was consistent with the model’s predicted finish time of between 1:15 PM and 1:39 PM based on a 3-4% delay, which resulted from patients not being ready; fortunately, they had already considered delay probabilities for the move which didn’t actually occur. Besides validating the move plan and predicting the final time, this model has several additional and important benefits. First, by finishing early, working hours were saved for the several dozen staff members involved in the move, as well as the staff at the new building who were asked to work a double shift. And UTMB now has the skeleton of an accurate model of their system that can be used in future situations.